Fill out my online form.
for Ford Focus Hatchback 3 2012 - 2017
Left Hand Drive & Right Hand Drive
Feature:
1,High-carbon steel memory steel sheet disperses pressure evenly to achieve seamless fit with the car windshield, achieve noise-free scraping test and keep the scraping test clear.
2,Made form pure nature rubber softer and more durable,surfaced teflon technology to prevent from aging quickly. Our wiper can against extreme cold snowstorm.
3. Deflector design based on aerodynamic principles. The vibration of the car wiper caused by wind force is eliminated.
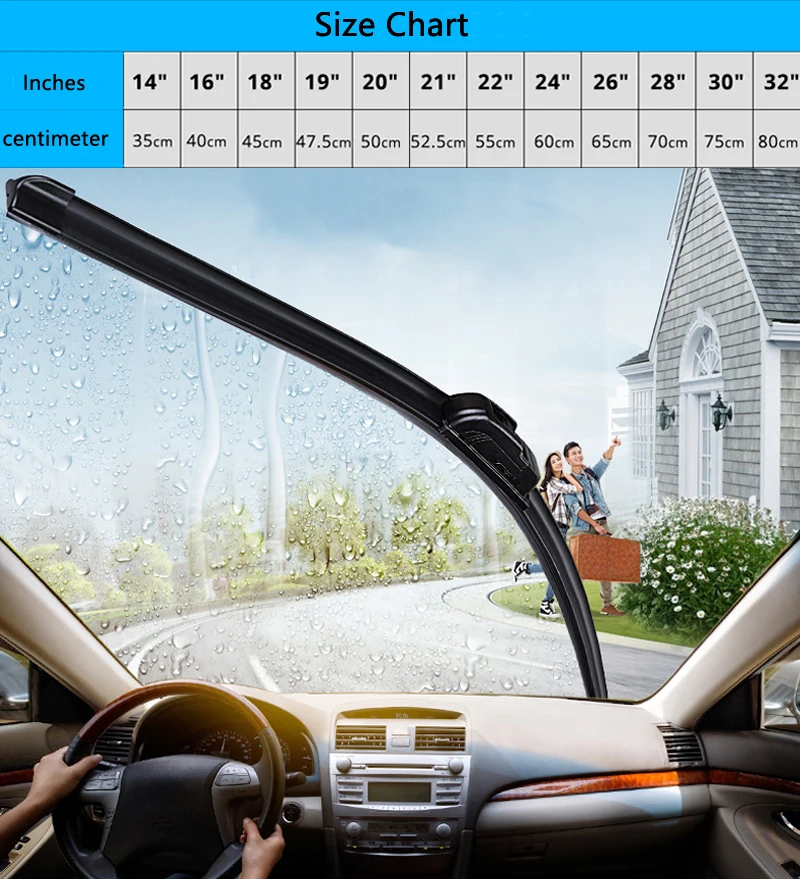
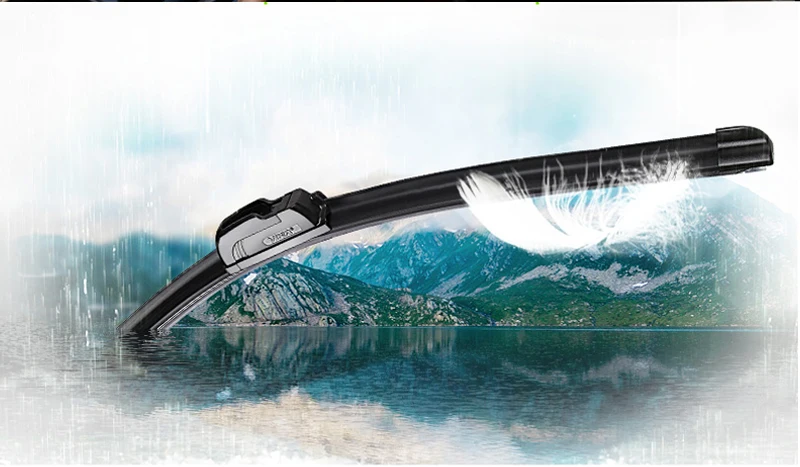



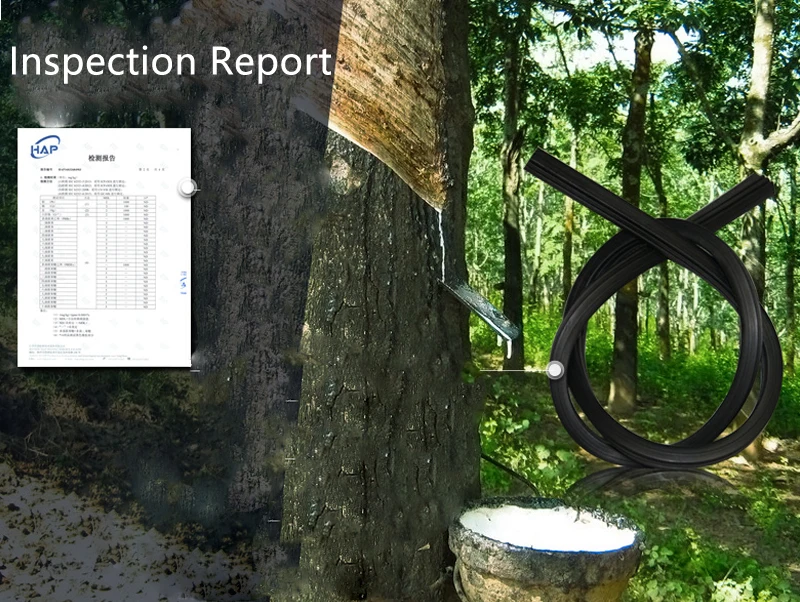
